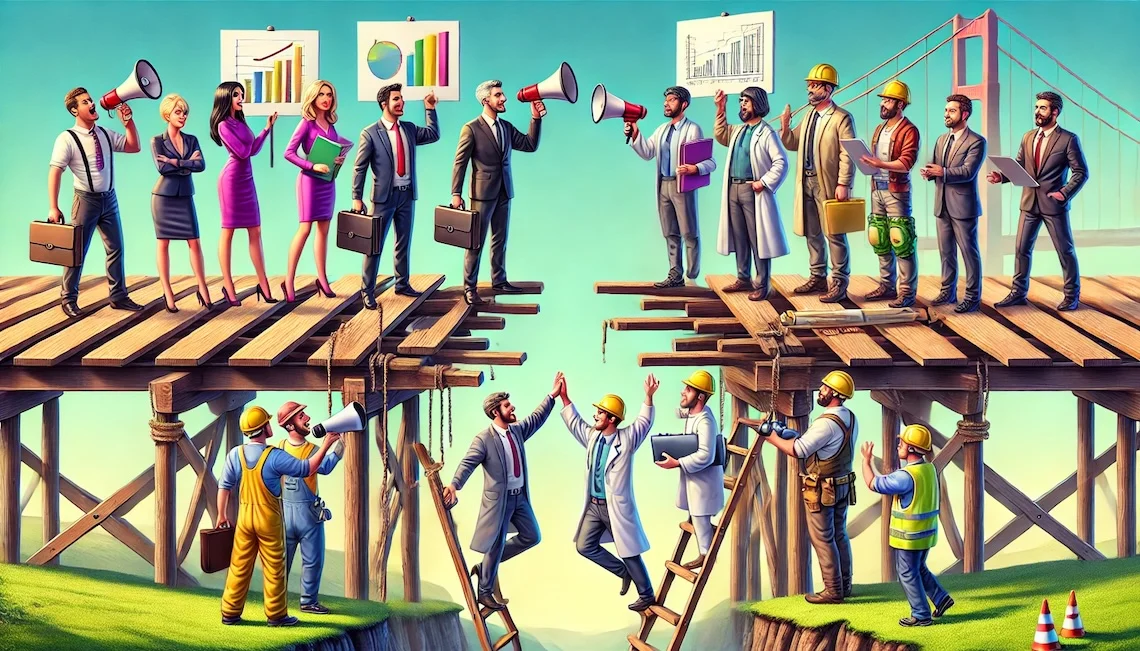
Florence Nightingale was a British nurse who was known and believed in data. She is considered the founder of modern nursing, as she was the first to apply the scientific method through statistics.
She made the history books of diagrams in 1858 with a publication related to epidemic deaths in the Crimean War.
Nightingale used a new form of visualisation, now named after her, to explain the causes of deaths and simple policies to prevent them. She succeeded in convincing policymakers and thus saved thousands of lives.
The data businesses are Netflix, Spotify, Airbnb, Apple, Microsoft, and Google. They bring value to their customers with the help of insights and lots of value.
Like Nightingale, they want to turn raw data into meaningful actions with a measurable impact.
So why is your company doing different?
“The goal is to turn data into information and information into insight,” said Carly Fiorina, former CEO of Hewlett-Packard.
Nightingale focused on raw data collection first; however, as you know now, the things that shifted everything were the visualisation and the storytelling.
She believed that tables don’t tell stories. No scientific man would ever even look into an appendix of a publication.
And all of that is simply by focusing on decision-making.
So this is for you, all those businesses, stubborn or not, that believe for them the right place to start is with decision-making and are willing to commit to this way.
Let’s explore the story of AirBnb, which started at this point, just like Zynga, focusing on data for decision-making. We’ll try to understand how companies pull this off and what pitfalls you must avoid.
The process that leadership teams make like this:
But you don’t have to do it in your little chamber, serving reports to someone. It is a team job; it is a cultural shift. Everyone must be involved in every decision.
You must create a cross-functional nature in the modern SMB because data scientists are only as valuable as the context; they must solve problems. The analysis must be embedded into the business and data sides, sitting with business leaders and engineers.
This shows one of the fundamental realisations of today’s business world: There are two huge gaps between the data creation, the people who curate data, and those who use it.
So, you must bridge these two gaps by putting all those functions into the same team, which is his definition of a cross-functional team.
Do something by hand as long as you can, and then start to automate the tiniest bit of it, repeat. The scientists are there to scale when speaking to every customer; making every decision by hand isn’t possible. Scientists are essential to scaling decision-making in a growing company without an ever-increasing headcount.
Making better, faster, more high-quality decisions is almost an afterthought to Airbnb. Airbnb focuses on making significant decisions by hand, collecting the best feedback from hosts, and only then automating it when this process becomes too painful.
If you want to take data-driven decisions seriously, you might provide a good role model. Most of their lessons are grounded in problems many companies have.
Florence Nightingale was a British nurse who was known and believed in data. She is considered the founder of modern nursing, as she was the first to apply the scientific method through statistics.
She made the history books of diagrams in 1858 with a publication related to epidemic deaths in the Crimean War.
Nightingale used a new form of visualisation, now named after her, to explain the causes of deaths and simple policies to prevent them. She succeeded in convincing policymakers and thus saved thousands of lives.
The data businesses are Netflix, Spotify, Airbnb, Apple, Microsoft, and Google. They bring value to their customers with the help of insights and lots of value.
Like Nightingale, they want to turn raw data into meaningful actions with a measurable impact.
So why is your company doing different?
“The goal is to turn data into information and information into insight,” said Carly Fiorina, former CEO of Hewlett-Packard.
Nightingale focused on raw data collection first; however, as you know now, the things that shifted everything were the visualisation and the storytelling.
She believed that tables don’t tell stories. No scientific man would ever even look into an appendix of a publication.
And all of that is simply by focusing on decision-making.
So this is for you, all those businesses, stubborn or not, that believe for them the right place to start is with decision-making and are willing to commit to this way.
Let’s explore the story of AirBnb, which started at this point, just like Zynga, focusing on data for decision-making. We’ll try to understand how companies pull this off and what pitfalls you must avoid.
The process that leadership teams make like this:
But you don’t have to do it in your little chamber, serving reports to someone. It is a team job; it is a cultural shift. Everyone must be involved in every decision.
You must create a cross-functional nature in the modern SMB because data scientists are only as valuable as the context; they must solve problems. The analysis must be embedded into the business and data sides, sitting with business leaders and engineers.
This shows one of the fundamental realisations of today’s business world: There are two huge gaps between the data creation, the people who curate data, and those who use it.
So, you must bridge these two gaps by putting all those functions into the same team, which is his definition of a cross-functional team.
Do something by hand as long as you can, and then start to automate the tiniest bit of it, repeat. The scientists are there to scale when speaking to every customer; making every decision by hand isn’t possible. Scientists are essential to scaling decision-making in a growing company without an ever-increasing headcount.
Making better, faster, more high-quality decisions is almost an afterthought to Airbnb. Airbnb focuses on making significant decisions by hand, collecting the best feedback from hosts, and only then automating it when this process becomes too painful.
If you want to take data-driven decisions seriously, you might provide a good role model. Most of their lessons are grounded in problems many companies have.